Material Informatics
Design of new descriptors
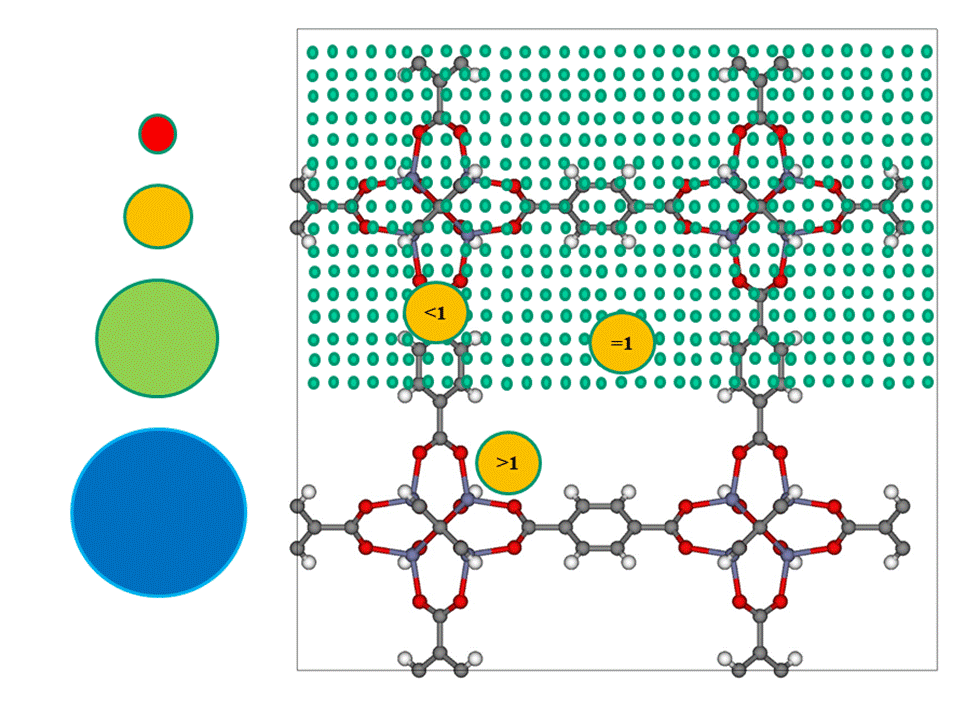
Aiming at both, the transferability of our model and the reduction of the training data set, we introduce 2 different classes of descriptors, based on fundamental chemical and physical properties: Atom Types [1] and Atom Probes [2] (figure). The main difference from previous models is that our descriptors are based on the chemical character of the atoms which consist the skeleton of the materials and not their general structural characteristics. With this bottom up approach we go one step down in the size of the descriptors employing chemical intuition.
References
[1] “A Universal Machine Learning Algorithm for Large Scale Screening of Materials”, G. S. Fanourgakis, K. Gkagkas, E. Tylianakis and G. Froudakis, Journal of the American Chemical Society 142 (8), 3814-3822 (2020)
[2] “A robust Machine Learning algorithm for the prediction of methane adsorption in nanoporous materials”, G. S. Fanourgakis, K. Gkagkas, E. Tylianakis, M. Klontzas, G. Froudakis, The Journal of Physical Chemistry A 123, 6080-6087 (2019)
Big data sets
In order to produce data for training our ML algorithm we performed High-Throughput GCMC simulations in the CoRE-MOF and CoRE-COF [1] data bases for many different gases in various thermodynamic conditions (H2, CH4, CO2, H2S, N2, H2O).
References
[1] CoRE (Computation-Ready, Experimental MOFs: Chem. Mater. 2014, 26, 21, 6185-6192).
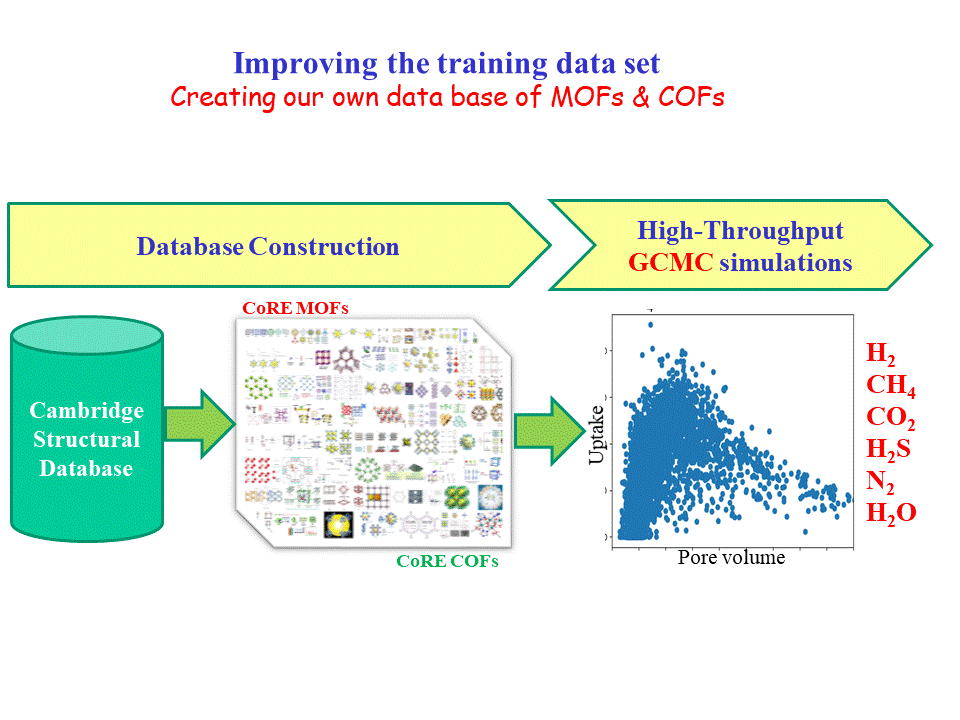
Training algorithms
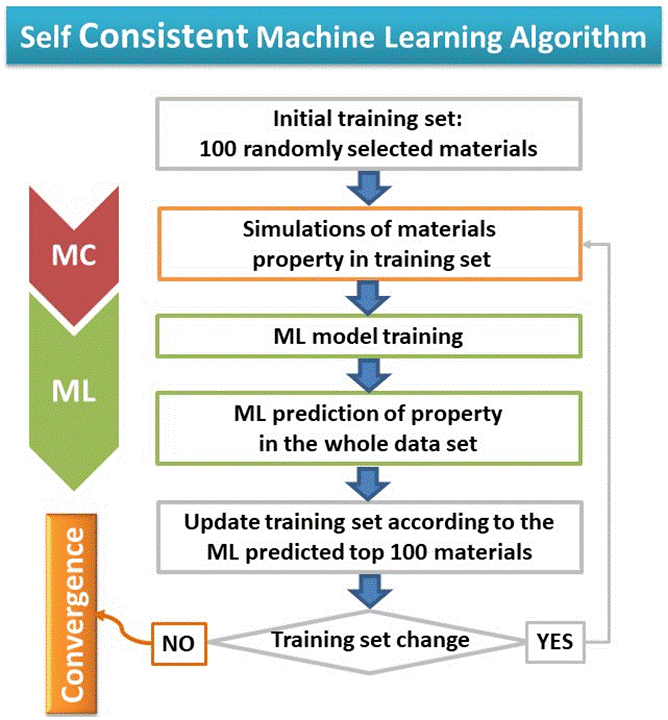
A novel training algorithm based on “Self-Consistency” (SC) replaced the standard procedure of linearly increasing of the TS (100, 200, 300, …). Our SC-ML methodology was tested in 5.000 experimentally made MOFs for investigating the storage of various gases (H2, CH4, CO2, H2S, H2O). For all gases examined, the use of both descriptors instead of building blocks leads to significantly more accurate predictions, while the number of MOFs needed for the training of the ML algorithm in order to achieve a specified accuracy can be reduced by an order of magnitude.